Images are not what they used to be. Or put a little more precisely, images are not for who they used to be for. Known for his in-depth investigations of contemporary state surveillance, the American artist Trevor Paglen has been focusing for the past several years on the rise of machine vision, an expanding realm of images made by machines for other machines, with humans often left out of the equation. This is the fast-developing world that, with artificial intelligence, is fuelling many of our current and most controversial innovations: from automated vehicles to robotics to facial recognition technology. Paglen is interested in exposing the inner workings of these often opaque technical systems and documenting a substratum of images that serve as the training material for computers learning how to see.
It is these image datasets, vast collections of labelled and categorised images used to teach computer vision systems to distinguish one object from another, that are the specific focus of two current Paglen exhibitions, one in London in the Barbican’s Curve gallery and one in Milan at the Osservatorio Fondazione Prada (the latter co-curated by the artist with Kate Crawford, co-founder of the AI Now Institute at NYU). The Barbican exhibition is dominated by a single newly commissioned work entitled From ‘Apple’ to ‘Anomaly’ – an array of approximately 30,000 printed photographs that virtually covers the sweeping wall of the Curve. The images are derived from ImageNet, arguably the most significant computer vision training set, first unveiled in 2009 by a team of AI researchers at Stanford and Princeton led by Professor Fei-Fei Li who once described the project as an attempt ‘to map out the entire world of objects’. A decade later, ImageNet consists of more than 14 million images, often scraped from online photo-sharing sites like Flickr and organised by hand by a crowdsourced army of workers into over 20,000 categories.
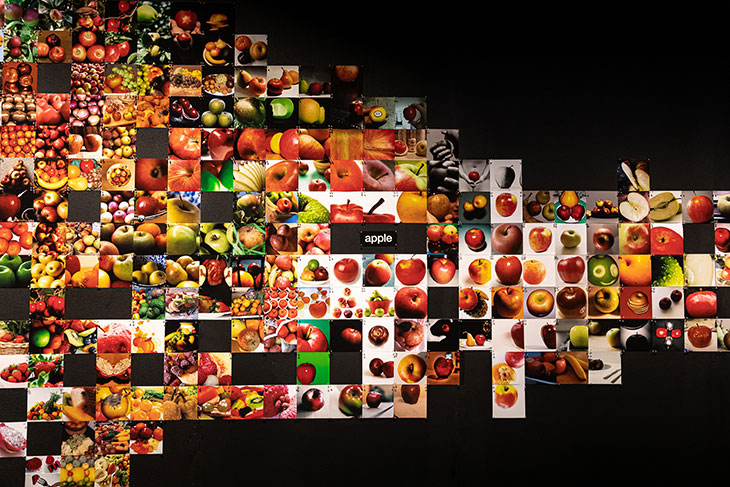
Installation view of ‘Trevor Paglen: From “Apple” to “Anomaly”’ at the Barbican, London, 2019. Photo: © Tim P. Whitby/Getty Images
For this work Paglen has chosen to display images belonging to a cross-section of these categories, beginning rather innocently with images clustered around the labels ‘apple,’ ‘apple tree’ and ‘fruit’, before moving on to more complex and contentious examples, from ‘minibar’ to ‘abattoir’ to ‘divorce lawyer’. For an exhibition about AI, this is a deliberately low-tech affair and it is striking to see these images, ones that seem to belong to the digital world, printed out here in physical form and pinned to the wall. The overall effect is that of a memory board in a college dorm room, blown up to the scale of all humanity.
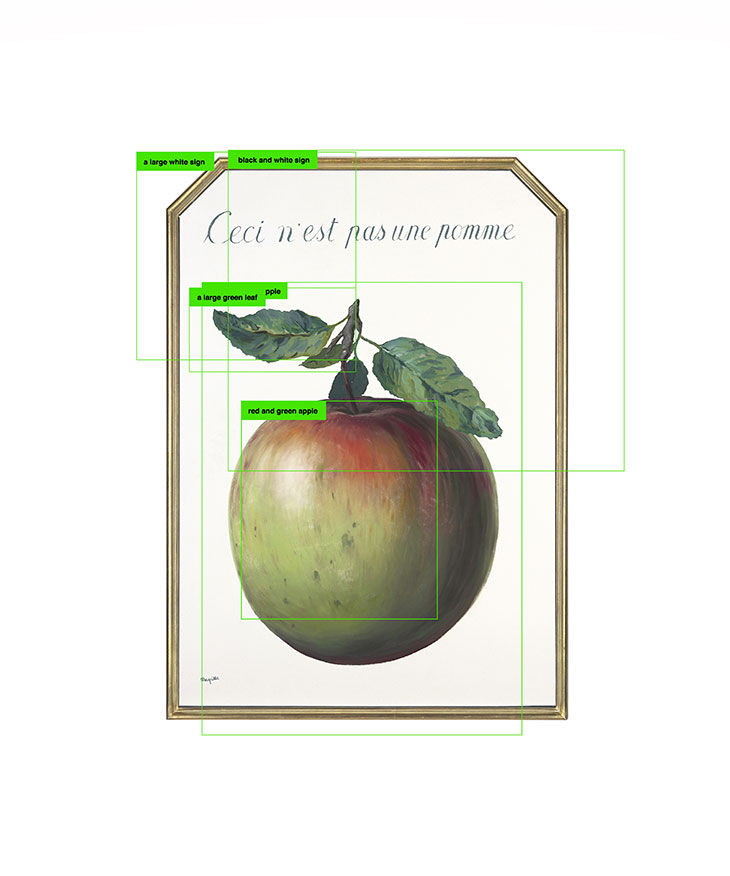
The Treachery of Object Recognition (2019), Trevor Paglen. Courtesy the artist, Metro Pictures, New York and Altman Siegel, San Francisco; © Trevor Paglen
It’s when people first appear among the photographs of objects that we begin to realise how strange and troubling this exercise in image classification really is. The category ‘picker’ includes smiling recreational strawberry pickers alongside Indian tea-leaf pickers and impoverished children picking through waste in a landfill. Any contextual distinction between these images is apparently flattened out in the eyes of the machine. Paglen’s goal here is to point out both the biases and absurdities contained within the organisational structure of ImageNet and to question what implications they may have for the AI systems that depend on such training sets. Predictably, for example, the labels ‘investor’, ‘entrepreneur’ and ‘venture capitalist’ present almost exclusively images of white, middle-aged men. Less flattering categories such as ‘selfish person’, ‘moneygrubber’ and ‘convict’ are considerably more diverse.
The ‘Training Humans’ exhibition at the Osservatorio Fondazione Prada provides a wider historical context to the phenomenon of AI-oriented image datasets. Paglen and Crawford have assembled 17 examples of training sets dating back to the 1960s, a still largely unknown genre of images they view as a form of ‘vernacular photography’. Presented mostly through print-outs of sample images from the datasets, the selections include early CIA-funded facial recognition research from 1963, the Multiple Encounter Dataset from 2011 composed of US police mugshots of multiple offenders, and the Japanese Female Facial Expression (JAFFE) database from 1997, a training set containing 213 images of 10 Japanese female models instructed to express anger, fear, disgust, surprise, joy and sadness. It amounts to an unsettling collection of human faces, with strong resonances of portraiture and 19th-century pseudoscience.
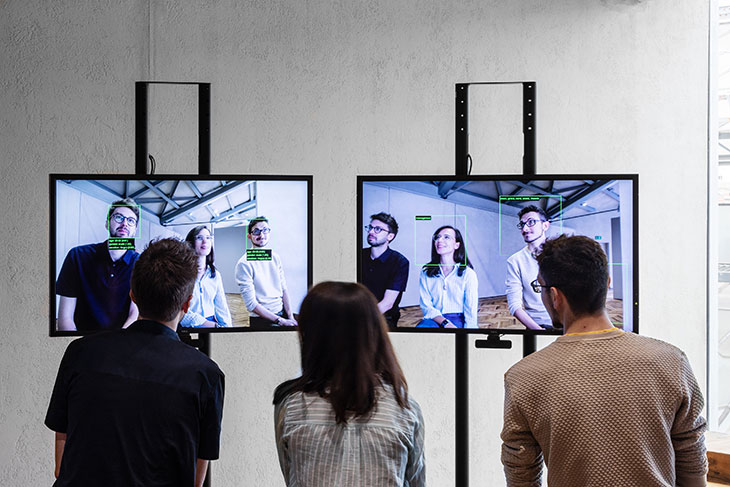
Installation view of ‘Kate Crawford, Trevor Paglen: Training Humans’ at Fondazione Prada, Milan, 2019. On the right-hand screen is Paglen’s ImageNet Roulette (2019). Photo: Marco Cappelletti; courtesy Fondazione Prada
ImageNet makes an appearance here as well. Most notably it serves as the training set for ImageNet Roulette, a computer vision system designed by Paglen that captures the video image of gallery visitors and assigns them labels from the ImageNet people categories (an online version of the work went viral last month). The labels are often uncomplimentary, gendered and even racist (my own image oscillated between ‘creep’ and ‘economist’), which Paglen and Crawford defend as a provocation to question the inherent prejudices of the ImageNet dataset and these forms of human categorisation more generally. In a text accompanying the exhibition they write that ‘ImageNet is an object lesson, if you will, in what happens when people are categorized as objects.’
‘Trevor Paglen: From “Apple” to “Anomaly”’ is at the Barbican, London, until 16 February 2020; ‘Kate Crawford, Trevor Paglen: Training Humans’ is at the Fondazione Prada, Milan, until 24 February 2020.
Unlimited access from just $16 every 3 months
Subscribe to get unlimited and exclusive access to the top art stories, interviews and exhibition reviews.
Are the art market’s problems being blown out of proportion?